2/14/2018
In a nondescript meeting room in a fluorescent-lit office park in Silicon Valley, a group of computer and planetary scientists were given the problem of deciding what to do with an asteroid on a collision course with Earth.
The group–split into teams representing different world leaders, scientists, and citizens with different ideas of how to deal with impending doom–proposed solutions to the theoretical threat. Role-playing wasn’t necessary, but a Brazilian astronomer did his best Russian leader impression and tried to cajole the others. A computer scientist designated as a planetary scientist gave his “expert” opinion on the threat of breaking up the asteroid into multiple asteroids.
Some wanted to use a rocket to nudge the asteroid, others wanted to nuke it and others wanted to collect more information. Issues like the path of the asteroid and what exactly it was made of further complicated the scenario. As in a Dungeons and Dragons game, a 20-sided dice was rolled to decide if the action was successful or not. Eventually, the asteroid approached and Japan looked like it was going to take a direct hit. The group decided to nuke the asteroid, saving Tokyo. The room erupted into cheers.
“This was like model UN but probably a little more fun,” one team member says.
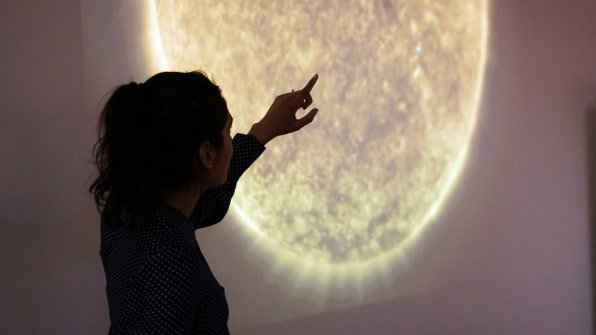
Silicon Valley hosts so many interns, research groups, and accelerators that many hotels are fully booked through the summer months. Only one accelerator in the Bay Area last summer, however, was comprised of a dozen planetary scientists and a dozen machine learning experts, strategizing ways to save us all from threats in space like asteroids, solar flares, and comets, and all within the space of eight weeks.
Frontier Development Lab, or FDL, is the brainchild of James Parr, a British space enthusiast and serial entrepreneur who saw an opportunity to bring more artificial intelligence and machine learning to space. He was inspired in 2013, when the Obama administration launched the Asteroid Grand Challenge to get more people working at new ways of finding asteroids, including those that could be mined for valuable resources and those that could destroy life on Earth.
“AI is the one thing that astronomers haven’t had the benefits of,” Parr says. “They just haven’t had time to work with it.”
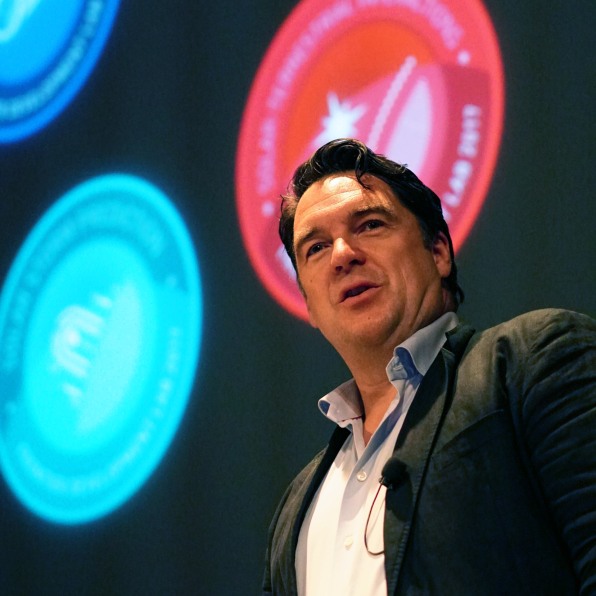
While the chance of a near-Earth object hitting the planet is distant, the stakes are existential. Sixty-five million years ago, a 10 trillion-ton asteroid hit the Yucatan Peninsula and killed 70% of life on Earth, including the dinosaurs. More recently, the meteor that exploded over Russia in 2013 caused a shock wave that smashed windows, damaged buildings, and injured 1,200 people. No one died, but if that same asteroid came in a little differently, at a more vertical angle, or in a more populated area, it could have been disastrous.
While scientists have known about these threats for decades, the extent of the risk wasn’t truly clear until recent years, as computers, better telescopes, and digital cameras drew it all into stark relief. “It was an ‘oh shit’ moment,” Parr says.
Those same technologies have also given scientists some tools to fight back. Bruce Willis’s mission in the movie Armageddon to blow up an asteroid with a nuclear weapon was not entirely made up (although, if you ask them, many planetary scientists think the plot of Deep Impact was more realistic). It’s an option.
After NASA launched its asteroid initiative, Parr approached the space agency to explore a new public-private initiative that would bring more of Silicon Valley’s AI talent to bear on the challenges of space. The world’s leading space agency is risk averse compared with today’s technology companies, and that prudent judgment means it rarely even lends its name out. But there is also an argument to be made that the more risky option would be to neglect what new technologies like AI could do to help humans survive a threat of extinction–and possibly even help us become a space-faring species.
After some cajoling, iterating, and fundraising, Parr finally got NASA on board and launched FDL in the summer of 2016 at the Mountain View, California-based headquarters of the SETI Institute, the nonprofit organization dedicated to the search for alien intelligence, with a class of 12 and a handful of mentors. Campers apply online–they’re often post-doc researchers in computer, data, and planetary sciences from across academia and the private sector–and those accepted receive a stipend and accommodations. Last summer’s researchers came from seven countries, companies like Apple and Hyperloop, and universities from Oxford to Cornell.
With a modest financial commitment from NASA, which provided only stipends for FDL’s U.S.-based participants in 2017, the camp relies heavily on private funding. So far, Parr says, finding companies that want to contribute funds and resources to the accelerator hasn’t been difficult. Its recent partners, who provide funding, cloud resources, and expertise, have included SpaceResources.lu, an initiative launched by the government of Luxembourg, as well as Lockheed Martin, IBM, Intel, KX, and Nvidia.
Private research into artificial intelligence has skyrocketed over the last few years with tech companies scrambling to hire AI researchers, or, at the very least, add terms like “deep learning” and “neural nets” to their business plans. An AI accelerator with the added benefit of a NASA partnership working on interesting problems attracted some attention.
And this, Parr points out, is a pretty interesting problem for just about everyone on Earth.
“For the first time in human history,” he says, “we had the ability to do something about an act of god. We don’t have to be victims.”
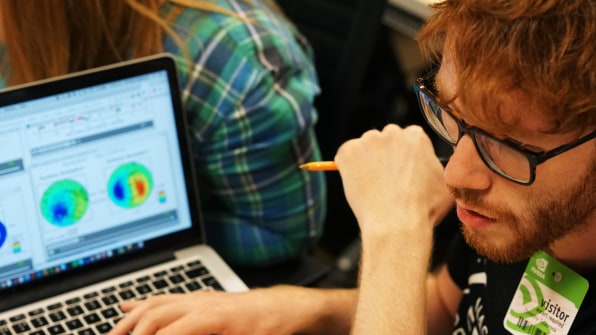
Extinction Level Events
The first near-Earth asteroid, or NEA, was identified in 1898, and another 500 or so were found in the succeeding century. (To be considered “near-Earth,” an asteroid or a comet must be on an orbit that brings it no further from Earth than the sun is to the Earth.) With new instruments, astronomers have since discovered a total of more than 17,000 NEAs. About half of the known NEAs have been found since 2010, and another 2,000 or so are discovered every year. One the size of the asteroid that hit Russia comes around about every three decades.
In 2005, lawmakers in the U.S. passed a congressional mandate: the country would find 90% of near-Earth objects are bigger than 140 meters, or 459 feet, by 2020. At the moment, astronomers know about almost all the near-Earth asteroids that are bigger than one kilometer in size, but it’s commonly thought that anything over 100 meters could be devastating if it were to hit a city. Current estimates put the number of those NEAs at hundreds of thousands to 5 million. Even if the actual number is at the lower end of that estimate, astronomers would have to increase their asteroid discovery rates by around a factor of 50 to get anywhere close to finding them all by 2020.
In 2013, NASA launched the Asteroid Grand Challenge to encourage scientists to “find all asteroid threats to human populations and know what to do about them”; in 2016, the U.S. opened a new office dedicated to planetary defense. NASA’s Jet Propulsion Laboratory also keeps a running list of asteroids that could possibly hit Earth in the next 100 years. The chances are often small, but some can get alarmingly large–a few have had a 1 in 1,000 chance of hitting Earth. Just this month, astronomers spotted an asteroid thought to be a bit larger than the one that hit Russia, and only a few days before it flew past us at a mere distance of 39,000 miles. (By comparison, the moon is 238,900 miles from Earth.)
A comet colliding with Earth is a similar but scarier threat for us. (There is some research that points to a comet and not an asteroid as the real dinosaur killer.) So-called short-period comets are on orbits that take their icy bodies on a short enough trip around the sun that the human race has had the technology to see them at some point on their orbit. Long-period comets–like Hale-Bopp in 1997–are more of a danger: They have orbital periods that take them past the sun every 200 to thousands of years. According to Kepler’s Third Law, celestial bodies on ellipsis move faster as they get closer to the sun. That means those far-away comets that we don’t know much if anything about speed up as they pass Jupiter on their way toward the sun, and the Earth.
“They sneak up on us,” says JL Galache, an FDL mentor and former consultant to NASA, who is the cofounder and CTO of Aten Engineering, an asteroid prospecting company.
Currently scientists can see a long-period comet approaching about six months to a year out. Comets are on average about 60 kilometers wide and, unlike asteroids, can come from any direction. It doesn’t help that these fast comets only reflect 3% to 4% of light, making them darker than coal and harder to track. Lucky for us, they are also pretty unlikely to hit Earth anytime soon. (In November, an 800-foot-long cigar-shaped rock that flew past the sun was initially assumed to be a comet, but was reclassified as an asteroid a week later; finally, the object, nicknamed Oumuamua, was designated as the first of a new class of interstellar object.)
A less severe but more probable event is a coronal mass ejection, or CME, a large explosion of plasma and magnetic field from the sun that fires a cloud of magnetized particles across space. When that cloud hits Earth’s magnetic field, it enhances auroras and causes electromagnetic fluctuations that could disrupt the electrical grid, blow transformers, and take out satellites.
Pilots would need to land planes without GPS. Astronauts in the International Space Station would be on their own. Depending on the severity, anything plugged in could be fried. So little is known about the effects since the largest one on record, the Carrington Event of 1859, was before the Industrial Revolution. What we do know is that it sent currents through telegraph cables, causing fires and shocking operators. A Carrington-like event today would cause damage costing between $600 billion and $2.6 trillion in America alone, according to a 2013 estimate by British insurance giant Lloyd’s. It could take weeks, if not months to get systems back online.
“They call that the trillion-dollar storm,” Parr says. Currently there is no surefire way to predict one.
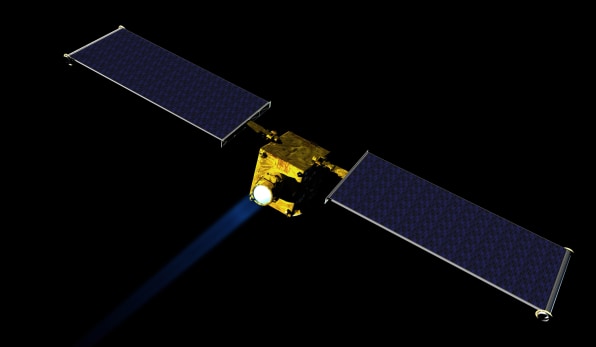
[Image: NASA/JHUAPL]
Defending Earth With Mountains Of Data
If we could predict that kind of storm, we could shut down the electric grid in advance. A better understanding of solar weather could also help reduce astronauts’ radiation exposure. As for dealing with a dangerous near-Earth object, blowing it up or nudging it out of its path is one possibility: Three near-Earth asteroids have already been visited by spacecraft, and NASA is currently planning testing of its first asteroid deflection mission. Another option is paint. Asteroids heat up from the sun and speed up, in a process called the Yarkovsky effect. By landing on an asteroid and painting it, the amount of sun the asteroid absorbs would be affected, thus altering its path. Comets could be dealt with in a similar way.
To design these solutions, scientists are turning to mountains of data. But space data is massive, multidimensional, and difficult or impossible to fully parse. Deep learning, on the other hand–part of a larger set of machine learning methods–promises to find patterns in large data sets and make connections that are unseen by humans.
Steve Chien, who leads the AI group at NASA’s Jet Propulsion Lab, argues that the data-digesting talents of deep learning aren’t just useful for designing autonomous robot explorers for the oceans believed to be on Jupiter’s moon Europa, for instance, but for finding signs of extraterrestrial life. “Unsupervised learning is extremely important to analyzing the unknown,” he told Scientific American. In December, a Google researcher and University of Texas astronomer announced that, after feeding NASA data into a machine learning algorithm, they had discovered two new planets that astronomers had missed in previous searches.
At the outset, FDL participants were split into teams to try to apply deep learning to five specific problems: how to model the shapes of NEAs, find water on the moon, learn more about mysterious long-period comets, get better at predicting space weather, and anticipate solar storms. The first week of the program was spent getting the computer scientists acquainted with space and catching planetary scientists up on the tools currently available in the AI field. Speakers at the top of the fields gave presentations, including Francois Chollet, who developed a deep neural network API called Keras that many of the teams were using.
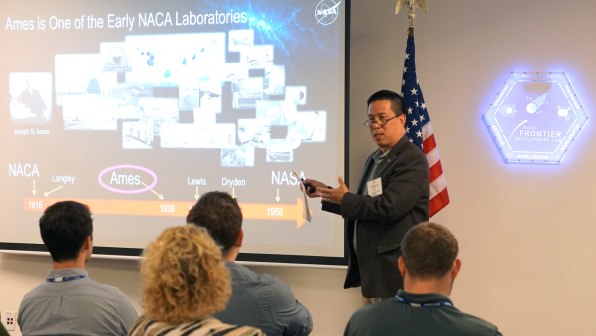
By the third week, all of the groups had a plan of attack for their projects and had received the go-ahead from their mentors. The team focused on notoriously hard-to-track long-period comets began by looking at their signatures. Comets have gas and dust tails that form the meteor showers we see on Earth. If you can record images or movies of those “shooting stars,” you can model their orbits and, in theory, obtain the orbit of the comet and where to look for it.
Already, projects around the globe are capturing thousands of images of the night sky each night to try to learn more about comets from these meteor showers. Currently, astronomers rely on data collected every night from projects like the EXOSS Citizen Science Project and the Cameras for Allsky Meteor Surveillance (CAMS), but because everything from fireflies to airplanes can look like meteors, all that noise has to be pulled out manually. The long-period comet team wanted to see if they could automate that process to reclaim time for more research.
The shape-modeling team, meanwhile, sought ways to use AI to map the surface of asteroids: To blow one up with a nuke or even nudge it away from Earth, scientists need good information on the dimensions and makeup of the thing. But photos of the NEAs are two-dimensional, low resolution, and don’t do a great job at capturing details of an already pretty dull object in the darkness of space.
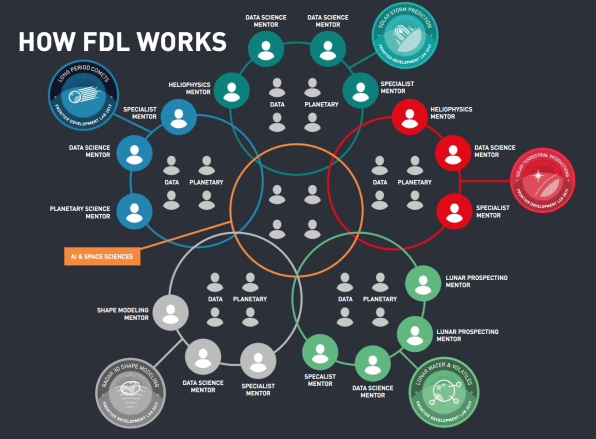
The campers weren’t only thinking about the risks of space. Asteroids are also valuable for future space exploration. The ability to extract water, and potentially minerals, for deep space flight is the only way to travel to Mars and beyond. Water, which is useful for drinking and can be broken down into the components needed for jet fuel, is pretty common in asteroids. In the best case, asteroids are 20% water. Not having to bring that water from Earth could save a lot of money: NASA estimates carrying water means $25,000 per gallon in fuel costs. If you priced water at $100 a liter, or about $378 a gallon, a 50-meter asteroid has about a billion dollars worth of water.
“They can kill us,” says Galache, the former NASA consultant. “But they are a source of resources that we are hopefully going to tap into.”
The lunar team was also examining ways to gain access to natural resources, like rare earth metals, silicates, and–most importantly for any future settlements–water. It’s likely that water is at the moon’s poles, where, out of the sun’s rays, it stays cold enough to not melt and float away with the moon’s lower gravity. First, though, you need to get to the poles, and that’s not easy: Communication devices need a direct line of sight to Earth, solar cells need a direct line of sight to the sun, and the terrain needs to be mapped so rovers don’t go careening into a crater.
On the last day, everyone gathered at Intel’s headquarters to present their results in front of peers from Google, NASA, Intel, and other research institutions. Equipped with lessons from a media training session earlier that week, the lunar water team opened up the presentations with a joke.
“We were wondering the best way to start a talk about lunar volatiles,” said Eleni Bohacek, a planetary scientist in the group. Her teammate, an AI researcher named Timothy Seabrook, had the answer. “First you have to break the ice.”
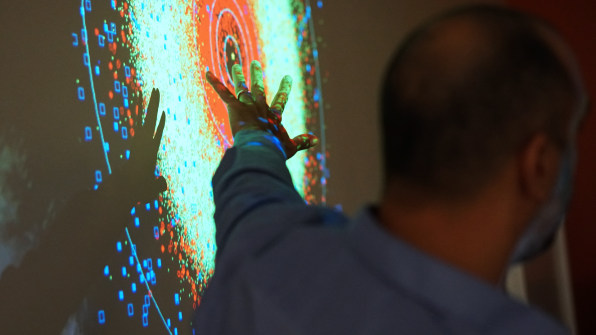
Their team showed that it could identify lunar craters with the deep learning algorithm they had used with 98% accuracy. Since 2011, 77 crater detection algorithms have been published, mostly done by hand and eye; none have come close to 98% accuracy.
“Twenty months ago that was kind of impossible,” says Parr.
These type of results are tracking closely with the rest of the AI community. “Achieving human level accuracy isn’t surprising anymore,” says Naveen Rao, the vice president and general manager of the Artificial Intelligence Products Group at Intel. “There is a forward progression.”
That forward progression means completing tasks with human-level accuracy in a fraction of the time it would take a human. The long-period comet team presented an automated and improved meteor classification with a system that takes a few minutes to get through 200,000 images and boasts a 90% accuracy rate. Their work even found a new meteor shower previously undetected. Someone in the audience actually gasped “wow.”
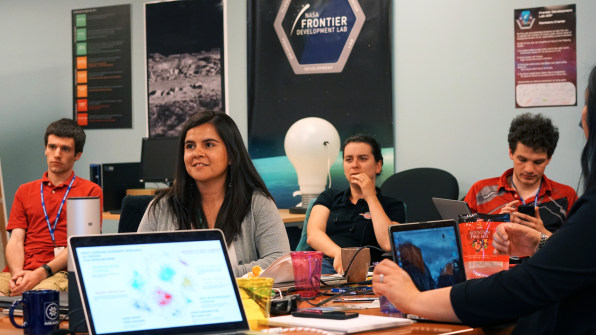
The team working on how to determine the shapes of asteroids showed they were able to speed up pre-processing of their data, figure out an asteroid’s spin state faster, and create synthetic models for their neural net to train on. With the help of a GPU-based cloud platform provided by IBM, the team reduced a modeling process that normally could take a week down to one to two hours.
The two teams looking at the sun also reported advances. The solar flare team used computer vision on photos of the sun to build a forecasting model that, though theoretical, marked an improvement over NOAA’s current prediction model: In theory it could warn stakeholders at least an hour out. The space weather team ingested multiple streams of data into their algorithm to determine important predictors of solar events. Scientists have thought that data from stations at the equator were crucial for figuring out solar events, and their algorithm backed that up.
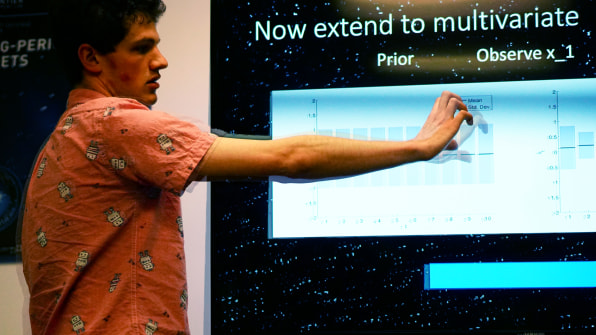
Still, for many of the planetary scientists, AI and deep learning, with their mysterious, sometimes black-boxed methods for coming up with results, is not ready to replace humans and physics just yet.
Burcu Kosar, a member on the space weather team and a physicist with NASA, says that some of the ways the algorithm was able to automate previously tedious data cleaning and labeling was a thrill. Computer scientists might be comfortable with an algorithm with a 98% accuracy rate even if they can’t explain how it got those results. Not for physicists.
That discomfort has helped fuel an emerging research area known as explainable artificial intelligence, or XAI. A growing number of AI researchers are warning against relying on systems that come up with outputs that are not easy to interpret. Human errors, biases, or assumptions could get baked in to the cryptic black boxes of AI systems without any way to double-check. NASA–again, with its safety-critical projects that send humans to space with billions of dollars of equipment–is unlikely to trust or rely on artificial intelligence until it’s easy to investigate why it’s coming to decisions.
Those concerns are also an argument for FDL’s interdisciplinary, public-private approach, educating researchers on the promises and limits of AI alongside the challenges of space. With FDL getting results within just eight weeks, its proponents insist that more resources and more time could result in some major advancements. And the participation of NASA researchers at FDL makes it a self-reinforcing mission.
Kosar, the NASA physicist who expressed reservations about AI, sees enough potential that she’s now on a committee that is beginning to explore ways to bring more machine learning into the space agency. “It’s a baby step in the giant leap that NASA will have to take,” she says. “This is going to be increasingly accepted in the scientific community.”
Bagikan Berita Ini
0 Response to "NASA's Silicon Valley Summer Camp Is Just Trying To Save Us From Space"
Post a Comment